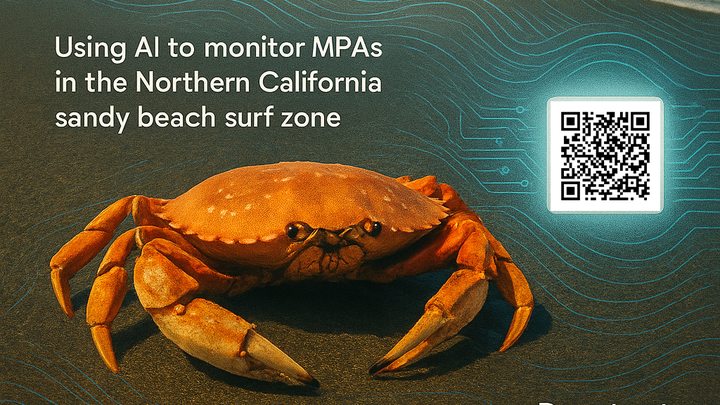
Pixels to Protection: Help fund AI in Marine Research
Donation protected
What is the context of this research?
Baited Remote Underwater Videos (BRUVs) consist of cameras mounted on an anchor, with a bait bag suspended at a distance to attract fish and marine species for video capture. This non-invasive, non-destructive method is ideal for monitoring fish species richness, diversity, and abundance, especially in Marine Protected Areas (MPAs), which are critical habitats for many species. While BRUV footage provides valuable insights, it can be time-consuming to analyze, as hours of video may yield only a few fish observations. To improve time efficiency, EventMeasure is working to develop an AI plugin called MaxN. MaxN automatically detects fish presence and timestamps each observation, allowing researchers to focus on key frames. This project aims to assess whether integrating MaxN into BRUV analysis will enhance time efficiency, increase consistency, and improve the capacity for cross-project comparison in MPA monitoring.
What is the significance of this project?
The ability to efficiently and accurately assess fish species richness, diversity, and abundance in Marine Protected Areas (MPAs) is critical for informed conservation and management. Traditional Baited Remote Underwater Video (BRUV) sampling, while non-invasive and highly effective, is hindered by the tedious and time-intensive process of analyzing extensive video footage. This project aims to revolutionize BRUV data analysis by integrating the AI program, MaxN, which automates fish detection and timestamping. This serves to enhance data processing efficiency, improve analytical consistency, and expand the capacity for large-scale, cross-project ecological assessments. If the goals of this project are met, this protocol could be implemented widely in marine research as a non-invasive and time-efficient method to monitor MPA success.
What are the goals of the project?
Our first goal is to explore the AI plugin MaxN as a viable alternative for manual BRUV video analysis. We will utilize the MaxN plugin with raw BRUV footage collected from an ongoing MPA monitoring effort. We will then evaluate MaxN by comparing the results of the manually processed BRUV footage with the results produced by AI, given the same footage. This comparison will assess whether AI can improve speed, accuracy, and scalability, ultimately reducing the time researchers spend analyzing footage.
Another key objective is to develop a protocol that enhances time efficiency, accuracy, and standardization. This protocol will establish a standardized method for integrating AI into BRUV analysis, improving accuracy, and ensuring consistency across MPA research. The goal is to create a replicable process that minimizes human error while maximizing time efficiency in data analysis.
Organizer
Katie Terhaar
Organizer
Trinidad, CA